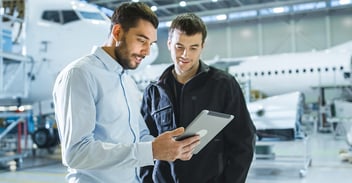
Dealing With AOG: Prediction Is The Key
Published :
Aviation industry loses approximately $6 billion every year due to AOG situation, the losses resulting primarily from non-availability of aircraft during AOG, causing lost revenues and increased expeditecost of parts and labor. All this while an estimated $2 billion worth of excess inventory sits in the value chain untapped – a classic case of dead inventory somewhere being worth its weight in gold elsewhere, but the twain never meets. While this may seem like an example of a simple demand-supply mismatch, there is a larger picture underneath. Given that we are talking about an industry with multiple stakeholders – OEMs (Aircraft and Engine Manufacturers and tier-1 suppliers), Airlines and Special Operators, third party MROs (Maintenance, Repair, & Overhaul) entities, Parts Distributors & Traders – the problem becomes quite complex.
In the next few paragraphs, I have attempted to breakdown the problem and corresponding solution into its individual components, list down enabling technologies, and present a holistic approach to addressing the AOG problem in the aviation industry. The good news is that there are solutions available in the industry for most of the sub problems.However, the challenge remains in the intent and the ability of larger stakeholders in the industry to come together to collaborate and address them.
What’s the Big Picture?
Traditionally, organizations have addressed the AOG problem only at the time and place when it occurs and by resorting to a reactive mode of parts search within the organization or in the ecosystem of parts distributors and go through a guessing game of parts availability, eventually incurring long delays and excessive fees.
To address the AOG problem in its entirety, one has to look holistically and way before the actual AOG occurs – by asking following questions:
Minimizing AOG Occurrence through predictive maintenance using AI/ML
Most of the AOG situations happen due to occurrence of “unscheduled events.” Most of the airlines and operators have mastered the art of forecasting scheduled maintenance using hours, cycles, and other combination of aircraft operational parameters., However, what throws them off is the unscheduled maintenance events. There is limited work done in this space, because it requires not only detailed analysis of historical data related to aircraft and parts usage, failure & replacement patterns, reliability calculations; but also sophisticated algorithms to create failure models for a part, for a given operating condition. Basically, answering the question – What’s the probability that a part will fail under a given operating condition after certain numbers of hours or cycles? Even with some of the most advanced forecasting algorithms, predicting unscheduled event has been a dream, primarily because of the enormity of variables involved. As per one estimate (according to a report in Aviation Week, Jan 18, 2016), “A single twin-engine aircraft with an average 12-hr. flight-time can produce up to 844 TB of data, and that’s just the engine, one engine”. This is where AI/ML comes to rescue. In fact, this enormous data (variables) which could be a bane for traditional manual forecasting model creation, can suddenly become the boon for AI/ML driven forecasting. AI/ML feasts on data, and there is no other organization (not even Facebook) that generates more data than an aircraft organization. With the vast amount of flight and maintenance data available for aircraft for decades, this becomes ground for AM/ML technology to munch through this data and create failure models for each part that no human brain can possibly create.
This unscheduled maintenance forecast when combined with the scheduled maintenance forecast can truly create a near accurate forecast – predictive forecast – that can help answer the above question. With this near accurate forecast, businesses can create proactive and optimal maintenance plan for parts so that the unscheduled failure events become few and far between, thereby minimizing AOG occurrences.
Better planning – through Inter Enterprise Collaboration
The concept and benefit of inter-enterprise visibility and collaboration is not new. This was demonstrated in the famous beer game of MIT in 60s, and had been adopted in many other industries, like high tech and retail, for more than a couple of decades. It’s a sad state of affair in the aviation industry where the “trust” level between OEMs, Airlines/Operators, and third-party MROs is quite low, which is a pre-requisite for such a collaboration.
In aviation industry what this concept translates into is: airlines sharing their maintenance forecast – at aircraft and at key component levels, like APU, Landing Gear, and Avionics with key OEMs and MROs and Parts Suppliers. This will help suppliers get better prepared for supporting airlines by providing capacity and parts availability in the event of an AOG. Airlines could strike a business arrangement and follow consignment or VMI model with suppliers in exchange for maintenance forecast. This model recently has been taking deep foothold within larger MROs/Part Distributors, who are able to pool the inventory across multiple airlines. This is a win-win for both the parties. Given that this model has been used in other industries for quite some time, the technology for sharing forecast already exists, with all alerts and workflows built around forecast exceptions. In late 90s, companies in high tech industry, like Dell, HP, Nokia, IBM had enabled a comprehensive inter-enterprise collaboration that spanned from long-term capacity collaboration, to mid-term forecast collaboration and near-term execution/pull signals. The fidelity of collaboration signals became clearer as the time closed in, and the corresponding contract agreements became stricter and binding. Aviation industry needs to follow this model, which will lead to better service levels (availability of parts during AOG) at lower cost that everyone can benefit from.
Better planning – through Better Inventory Positioning and policy optimization
Once better forecast and inter-enterprise collaboration is enabled, they become the primary ingredient for optimal inventory positioning and stocking policy definition for each location. While this part of the puzzle has been largely solved and there are sophisticated tools in the market that can help with the inventory planning & optimization, it’s important to keep certain nuances of the aviation industry in mind. For example, given the high cost of AOG in most cases, the inventory should be as much forward stocked as possible, and also the SLA should be considered at high level, even if it means higher inventory stocking levels and associated cost.
There is one other challenge that needs to be addressed, which is the long tail of parts that are not frequently replaced or repaired, which could cause significant downtime for aircraft if they were to fail, given the large lead time for procurement. By the very nature of their low utilization, they have small historical data to be useful for any AI/ML type forecasting. However, when looked across multiple airlines – by someone like an Aircraft OEM or a third-party aggregator – suddenly the critical mass of data is available to do a meaningful forecast. Role of an OEM as an anonymized aggregator and forecaster of data is extremely critical for AOG planning. The challenge lies in trust and leverage. I personally feel a third party aggregator and forecaster could add immense value for long tail forecasting.
Minimizing Aircraft Downtime through Real-Time Inter-Enterprise Parts Visibility
This last step of the framework tackles the basics of the problem. Even with all the AI/ML driven predictive maintenance, inter-enterprise collaboration, and advanced inventory optimization, AOG can and will happen. The question is what structures need to be in place, so that when it does happen, organization can minimize the aircraft downtime.
Today when AOG happens, a series of events unfold from checking of parts availability within internal warehouses (which is also limited), to looking into external parts distributors’ portals, to scouring the various parts exchange platforms like ILS, Partsbase, Plane etc. While parts exchanges tries to solve this problem, there are two key issues with them:
The approach is fundamentally flawed. What is required is a near real time visibility into parts availability by checking the database of a parts supplier directly through a real time mechanism like an API call. This check could be done not just with one part supplier, but with many, and thereby retaining the competitive nature of the business. Airlines with AOG then could secure the part with best price and availability through a real time B2B transaction.
Taking the AOG occurrence situation to the next level, where in many instances the discrepancy is identified during the flight itself before landing. If there was a way to electronically transmit this information to the ground staff ,the technicians, so that they could be ready with the right part at the right time when the aircraft lands, it could provide a huge leg up on minimizing AOG related ground time. This of course requires connectivity between flight ops systems and maintenance and inventory system – not just technical connectivity, but also business process level workflow that can drive the parts request, issue, shipment, use, and return – all seamlessly across the three systems.
Summary and Call to Action
So, in summary, there are multiple levels – from forecasting to collaboration to planning to execution – that have to be orchestrated by an organization to truly tackle the AOG situation end to end, and way before it occurs. It will also be clear to a discerning reader that addressing AOG in aviation industry requires multiple stakeholders and systems working together cutting across organizational boundaries. Easier said than done. As has been done in other industries, specifically retail and high tech, there is a need for “Ecosystem Stewards” to emerge from leading OEMs, Airlines, and third-party MROs, to form a consortium to look at this problem holistically, and institute structures, policies, and use of latest technologies to help address this menace.
All Rights Reserved. © Copyright 2024. Ramco Systems.